We've joined our RTI Health Solutions colleagues under the RTI Health Solutions brand to offer an expanded set of research and consulting services.
Natural Language Processing Offers Solution For Low SDoH Documentation
As growing evidence shows strong associations between non-clinical factors and clinical outcomes, providers and academics have developed a significant interest in social determinants of health. Despite the urgent need for data related to SDoH, there are numerous barriers that make widespread data collection sparse.
At the 2022 ONC Virtual Tech Forum, several researchers discussed the challenges to collecting SDoH information in clinical notes and how physicians might be able to adapt and coordinate to boost data collection efforts. The session also reviewed how Natural Language Processing (NLP) can facilitate data extraction from electronic health record (EHR) systems in support of public health.
SDoH data collection methods are inconsistent
SDoH metrics are helpful to understand society at both the population and individual levels. Currently, physicians can report their SDoH data collections to public health agencies (PHAs), such as local and state health departments, the Occupational Safety and Health Administration or the Centers for Disease Control. This data sharing helps PHAs to identify which communities and patients are most at risk.
Unfortunately, the collection of SDoH is not standardized or mandatory. This means the rate at which data are collected and the kind of data that is documented are inconsistent across county, state, health plan, provider or other divisions of care.
Most common barriers to clear, centralized SDoH data
One of the first steps to aggregating SDoH data is to define it, and there are varying definitions of what social determinants of health encompass.
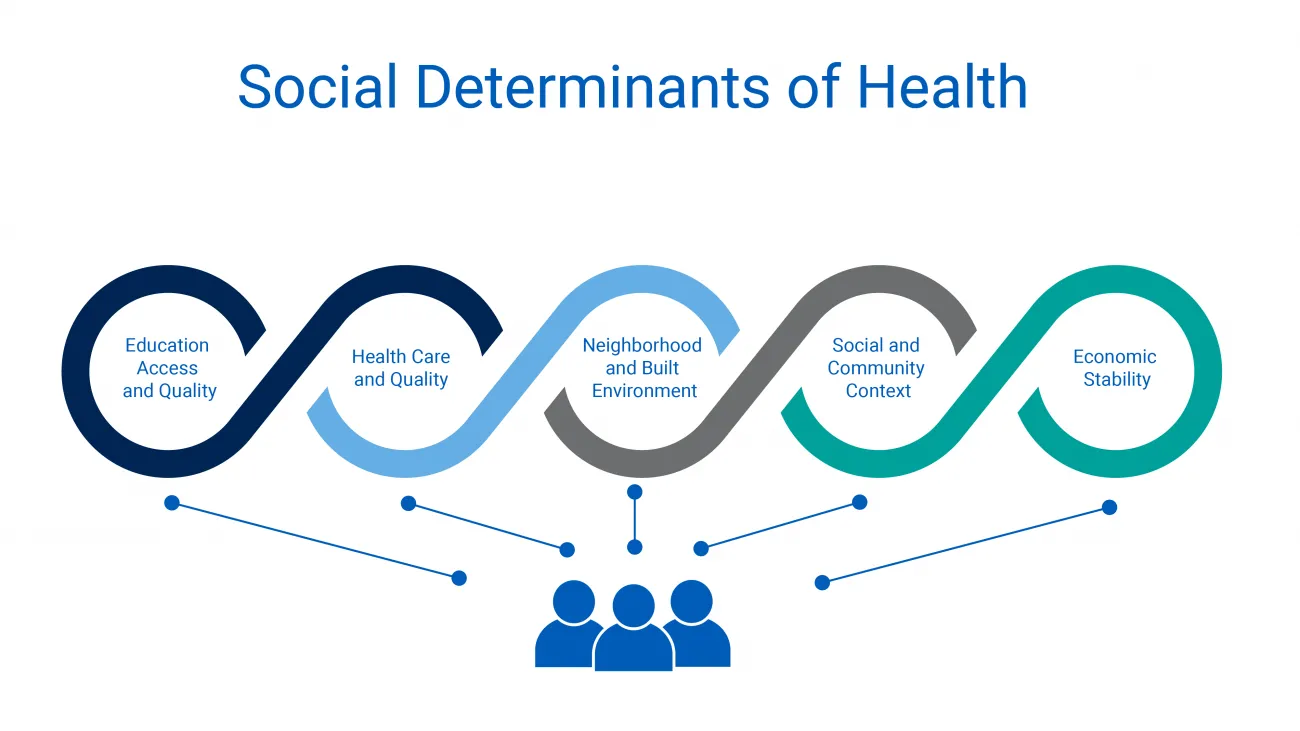
Most clinicians agree that SDoH should be documented in a patient's EHR as part of the patient intake process. Clinicians may discuss these topics with their patients to gain more salient details needed to offer targeted advice. However, documentation of these findings in clinical notes varies greatly and is generally inconsistent.
Patient/provider reluctance
Primary care providers are generally the clinicians tasked with documenting and collecting SDoH information. While providers may talk about issues such as housing insecurity or insurance with a patient, they express that such conversations are difficult when few resources exist to remedy the problem, or when the provider isn't in a position to directly affect change. Patients are also reluctant to share details about issues for which lived experience suggests there is little likelihood for change.
Prior discrimination
BIPOC patients who have experiences with discrimination or racism are often hesitant to disclose information or document personal details with healthcare providers. Structural racism in medicine has created medical mistrust that impacts a population's willingness to share personal information and struggles.
Lack of collection and reporting standards
Because there is no standardized method for collecting social and behavioral determinants from patients, these data are documented infrequently and in myriad ways. The most common SDoH documented is race and preferred language (at rates of 98% and 50%, respectively). However, additional SDoH factors are documented much less, with fewer than 2% in many cases and peaking at 4% for others.
Providers also cite difficulty or inability to document SDoH as the individual's social needs are often complex and more nuanced than available SDoH codes or selections in EHRs. Creating a standardized method for data collection may not be reasonably attainable or equitable, since culturally competent care is not one-size-fits-all.
EHR can bridge the gap in data collection efforts
A 2019 National Electronic Health Records Survey study exploring the data exchange between provider reporting and collection capabilities found that recording behavioral and social DoH was higher among physicians with public health reporting capabilities. Physicians who electronically exchanged patient health information with other providers were more likely to electronically record behavioral determinants of health and SDoH.
The real-world data most commonly used by clinicians are: EHR, linked mother-baby data, tumor registries, and healthcare claims data.
Natural language processing to extract SDoH
Natural language processing (NLP) is an AI tool giving computers the ability to understand text and spoken words. OneFlorida, a partnership of Florida-based healthcare entities that includes academic health centers, used NLP to better understand its populations.
The software was able to take existing EHR and extract seven categories of SDoH (each with subclasses) based on keywords found in clinical notes:
- Socio-economic
- Education
- Social and community context
- Health and healthcare
- Neighborhood and physical environment
- Gender, race and ethnicity
- Veteran status
Digital health innovations such as NLP have the potential to address many of the barriers that exist in achieving necessary data sets on historically under resourced populations.
Extract SDoH data from your patient populations
As healthcare data becomes more relevant to health systems looking to solve inequities in healthcare and achieve value-based care, understanding how to reach patients and improve documentation efforts is vital. Talk with one of our consultants today about your current data collection processes and how RTI Health Advance can support your goals.
—
Panelists for the ONC Tech Forum session included: Dr. Catherine DesRoches of Harvard Medical School/Beth Israel Deaconess Medical Center, Dr. Elizabeth Shenkman of the University of Florida, Dr. Chelsea Richwine of ONC, Dr. Yonghui Wu of University of Florida, and moderator JaWanna Henry of Health IT.
Subscribe Now
Stay up-to-date on our latest thinking. Subscribe to receive blog updates via email.
By submitting this form, I consent to use of my personal information in accordance with the Privacy Policy.